Workshops
The 10th International Workshop on Security and Privacy in Big Data (BigSecurity 2022)
Big Data Security 2
On the detection of adversarial attacks through reliable AI
Ivan Vaccari (National Research Council, Italy); Alberto Carlevaro (University of Genoa & CNR-IEIIT, Italy); Sara Narteni (Consiglio Nazionale delle Ricerche, Italy); Enrico Cambiaso (National Research Council, CNR-IEIIT, Italy); Maurizio Mongelli (National Research Council of Italy, Italy)
Overlapped Connected Dominating Set for Big Data Security
Li Yi (Shanghai Institute of Microsystem and Information Technology Chinese Academy of Sciences, China); Weidong Fang (Key Laboratory of Wireless Sensor Network & Communication, SIMIT, CAS., China); Wei Chen (China University of Mining and Technology(CUMT), China); Wuxiong Zhang (Shanghai Institute of Micro-system and Information Technology); Guoqing Jia (Qinghai University for Nationalities, China)
SCSGuard: Deep Scam Detection for Ethereum Smart Contracts
Huiwen Hu, Qianlan Bai and Yuedong Xu (Fudan University, China)
When Wireless Federated Learning Meets Physical Layer Security: The Fundamental Limits
Honan Zhang (Southwest Jiaotong University, China); Chuanchuan Yang (State Key Laboratory of Advanced Optical Communication Systems and Networks, Peking University, China); Bin Dai (Southwest Jiaotong University, China)
WMDefense: Using Watermark to Defense Byzantine Attacks in Federated Learning
Xu Zheng, Qihao Dong and Anmin Fu (Nanjing University of Science and Technology, China)
Session Chair
Qiang Ye (Memorial University of Newfoundland, Canada)
Big Data Security 1
Automatic Selection Attacks Framework for Hard Label Black-Box Models
Xiaolei Liu and Xiaoyu Li (University of Electronic Science and Technology of China, China); Desheng Zheng (Southwest Petroleum University, China); Jiayu Bai and Yu Peng (University of Electronic Science and Technology of China, China); Shibin Zhang (Chengdu University of Information Technology, China)
Interpretability Evaluation of Botnet Detection Model based on Graph Neural Network
Xiaolin Zhu and Yong Zhang (Beijing University of Posts and Telecommunications, China); Zhao Zhang (Beijing University of Posts and Telecommunication, China); Guo Da, Qi Li and Zhao Li (Beijing University of Posts and Telecommunications, China)
A Generative Model for Evasion Attacks in Smart Grid
Venkata Praveen Kumar Madhavarapu (Missouri University of Science and Technology, USA); Shameek Bhattacharjee (Western Michigan University, USA); Sajal K. Das (Missouri University of Science and Technology, USA)
IoT Botnet Detection framework from Network Behavior based on Extreme Learning Machine
Nasimul Hasan, Zhenxiang Chen, Chuan Zhao, Yuhui Zhu and Cong Liu (University of Jinan, China)
Learning Based and Physical-layer Assisted Secure Computation Offloading in Vehicular Spectrum Sharing Networks
Ying Ju and Yuchao Chen (Xidian University, China); Haoyu Wang (University of California Irvine, USA); Lei Liu, Qingqi Pei and Zhiwei Cao (Xidian University, China); Neeraj Kumar (Thapar University Patiala, India)
Session Chair
Yuedong Xu (Fudan University, P.R. China)
Big Data Privacy 1
FedTSE: Low-Cost Federated Learning for Privacy-Preserved Traffic State Estimation in IoV
Xiaoming Yuan and Jiahui Chen (Northeastern University, China); Ning Zhang (University of Windsor, Canada); Chunsheng Zhu (Southern University of Science and Technology, China); Qiang Ye (Memorial University of Newfoundland, Canada); Sherman Shen (University of Waterloo, Canada)
FedCluster: A Federated Learning Framework for Cross-Device Private ECG Classification
Daoqin Lin, Yuchun Guo, Huan Sun and Yishuai Chen (Beijing Jiaotong University, China)
of different clients is extremely non-independent and identically distributed(Non-IID) as a client has only 1 or 2 classes of the total 5 classes of heartbeats. Subset data sharing was proposed to compensate for Non-IID data in image tasks, but the original federated learning algorithm FedAVG with such shared data still performs bad, especially for the rare type, as the parameters
from different clients to server are treated equally. Based on our observation that clients can be clustered in terms of their ECG data, we propose a novel parameter update framework, named FedCluster, to improve the federated model to diagnose rare types of clients. To make FedCluster work, we cluster the parameters submitted by different clients to the server to transfer knowledge between similar clients, especially those of rare types, and we propose a hierarchical method for obtaining global shared data suitable for unbalanced ECG data in extreme distribution. Considering the practicality of the proposed method, we only select modified-Lead II of MIT-BIH database to verify our method. The experimental results show that, compared with original FedAVG, 1) our method improves the overall classification accuracy by 6.14%; 2) the recognition accuracy has been improved by 89.38%, using client with high skewness data distribution such as client 232.
Incentive and Knowledge Distillation Based Federated Learning for Cross-Silo Applications
Beibei Li, Yaxin Shi and Yuqing Guo (Sichuan University, China); Qinglei Kong (The Chinese University of Hong Kong, Shenzhen, China); Yukun Jiang (Sichuan University, China)
Novel Efficient Block Chain and Rule-based Intelligent Privacy Share System in Future Network
Xiaolong Deng (Beijing University of Post and Telecommunications, China); Tiejun Lv (Beijing University of Posts and Telecommunications, China); LinMing Song (Beijing University of Post and Telecommunications, China)
Hierarchical Attention Network for Interpretable and Fine-Grained Vulnerability Detection
Mianxue Gu (Hainan University, China); Hantao Feng (XIDIAN University & National Computer Network Intrusion Protection Center, China); Hongyu Sun (Xidian University, China); Peng Liu (Pennsylvania State University, USA); Qiuling Yue (Hainan University, China); Jinglu Hu (Waseda University, Japan); Chunjie Cao (Hainan University, China); Zhang Yuqing (University of Chinese Academy of Sciences, China)
Session Chair
Ning Zhang (University of Windsor, Canada)
Big Data Privacy 2
DRL-based Optimization of Privacy Protection and Computation Performance in MEC Computation Offloading
Zhengjun Gao and Guowen Wu (Donghua University, China); Yizhou Shen (Cardiff University, United Kingdom (Great Britain)); Hong Zhang (Donghua University, China); Shigen Shen (Shaoxing University, China); Qiying Cao (Donghua University, China)
PRESSGenDB: PRivacy-prEserving Substring Search on Encrypted Genomic DataBase
Sara Jafarbeiki and Amin Sakzad (Monash University, Australia); Shabnam Kasra Kermanshahi (RMIT University, Australia); Ron Steinfeld (Monash University, Australia); Raj Gaire (Data61|CSIRO, Australia)
Efficient and Privacy-Preserving Logistic Regression Scheme based on Leveled Fully Homomorphic Encryption
Xin Zhao, Chengjin Liu, Zoe Jiang and Qian Chen (Harbin Institute of Technology, Shenzhen, China); Junbin Fang (Jinan University, China); Daojing He (East China Normal University, China); Jun Zhang (Shenzhen University, China); Xuan Wang (Harbin Institute of Technology Shenzhen Graduate School, China)
Machine and Deep Learning Approaches for IoT Attack Classification
Alfredo Nascita, Francesco Cerasuolo, Davide Di Monda, Jonas Thern Aberia Garcia, Antonio Montieri and Antonio Pescapé (University of Napoli Federico II, Italy)
Phishing Attack Detection with ML-Based Siamese Empowered ORB Logo Recognition and IP Mapper
Manish Bhurtel, Yuba Siwakoti and Danda B. Rawat (Howard University, USA)
Session Chair
Youyang Qu (Deakin University, Australia)
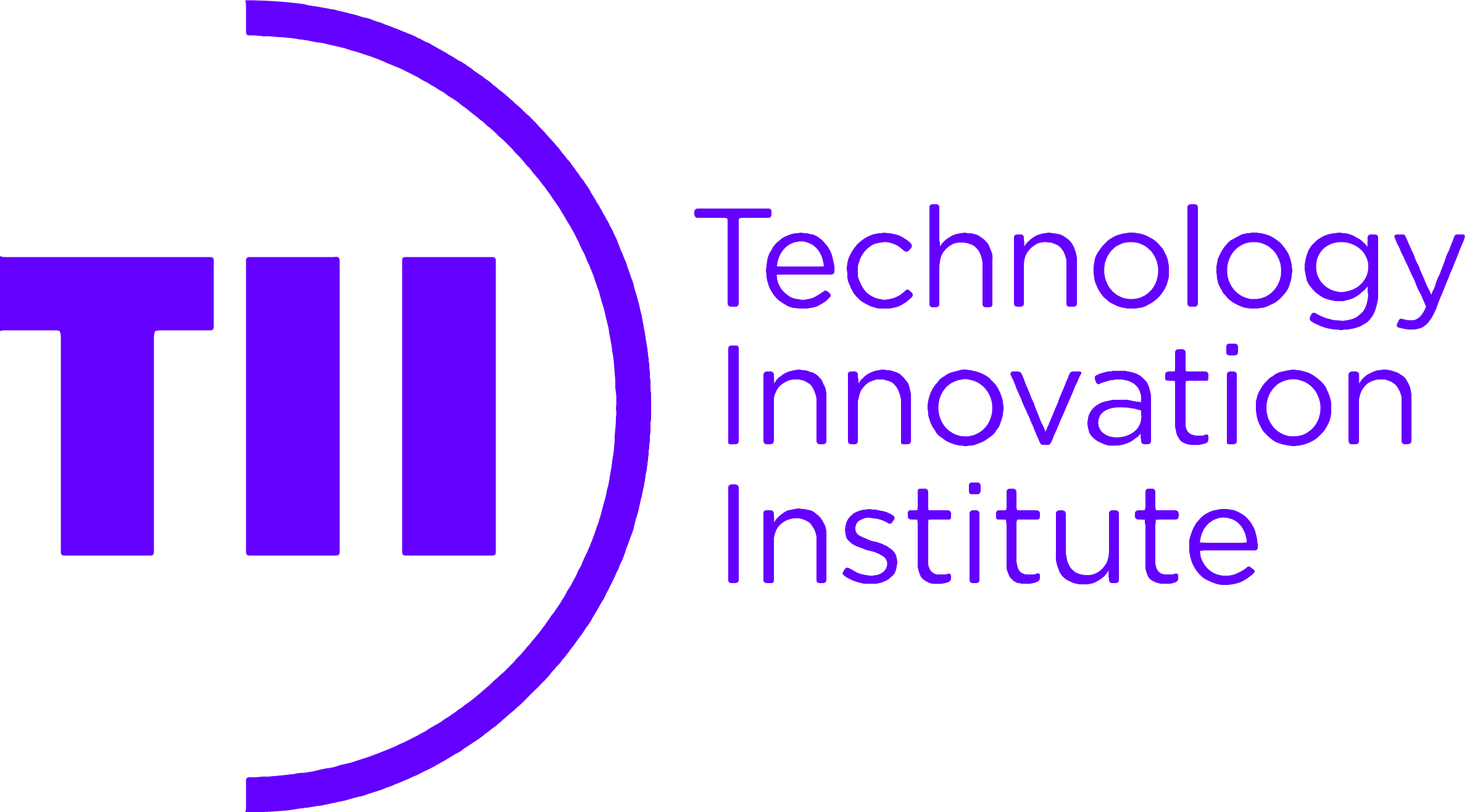
Made with in Toronto · Privacy Policy · INFOCOM 2020 · INFOCOM 2021 · © 2022 Duetone Corp.